My Account Details
Three Levels of Learning Analytics in Adaptive Learning
How does adaptive technology use data science to personalize the learning experience?
How does adaptive technology use data science to personalize the learning experience?
A lot of people talk about analytics, but what does it really mean and how does it apply to the science of learning? At McGraw-Hill we look at the world of learning analytics as being broken into three separate levels – each of which is defined by how learner performance data is used. Here’s a look at the three-stage framework we use to illustrate three key levels of learning analytics.
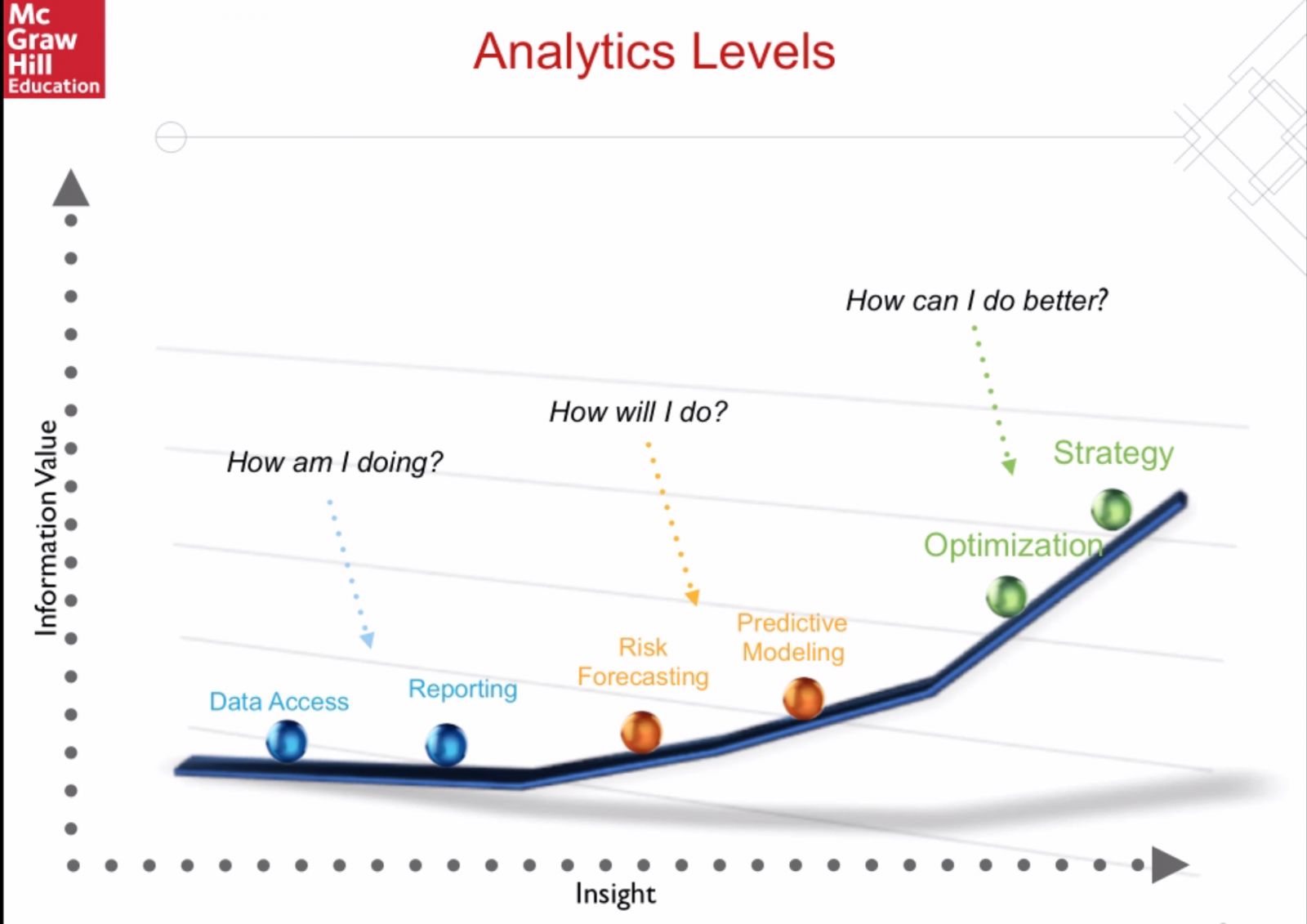
1. Data about the past - Key aspects of this first level of analytics include data access and reporting. Similar to having a rear-view mirror in a car, this data can help us understand the past knowledge and activities that resulted in the current knowledge state for students. It can tell us "how is this learner doing today?" and "how did this learner get here?" By gaining a deep understanding the decisions and habits that cause learners to arrive at their current knowledge states, we can better predict what they will need (e.g.types of content; learning tools) to learn effectively in the future.
2. Data that forecasts the future - New techniques such as predictive modeling and risk forecasting provide data to inform future learner responses and decisions. It helps us gain learner insights to predict performance habits and outcomes. At the McGraw-Hill Digital Platform Group we have a large team of analysts dedicated to the science of using technology and learner data for forecasting. We want to understand not only how learners arrived at their current knowledge states, but how future decisions and learning paths might impact their learning experience.
3. Optimization - The top tier or "Holy Grail" of learning analytics is not so much data itself as the practical application of data to help learners achieve a desired state. Insights and findings from the first two levels of learning analytics are applied to create optimal personalized learning pathways. Each learner is likely to make a very different set of decisions and knowledge points as they progress toward an optimal learning path. Our goal is to use data and analytics to equip students and instructors with information and tools they need to efficiently achieve optimal personalized learning pathways.
So how does learning analytics combine with adaptive learning technology to truly impact learning? We believe that discovering the optimal path for each learner requires the best of machine intelligence (adaptive learning) and empowered human intelligence supported by data-driven insights or visualizations (learning analytics). The primary goal of our approach to learning analytics is to empower students, teachers, parents and administrators with the knowledge and efficient technology tools they all need to help learners achieve desired outcomes.
We believe that empowering instructors and students with the very best of adaptive technology informed by deep learning analytics creates an unbeatable combination to achieve desired educational outcomes.
About the Author
Al Essa
- Vice President , Research and Analytics
Al Essa is vice president of Data Sciences. He leads the scientific and engineering efforts that embed a data-driven approach to teaching and learning throughout McGraw-Hill's digital product portfolio.